UTHealth Houston wins $6.4M NIH grant to develop deep learning model for Alzheimer’s
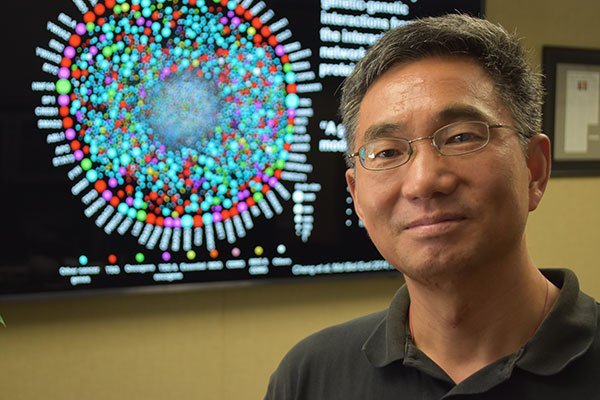
UTHealth Houston has been granted a $6.4 million fund by the National Institute on Aging for the next five years. The fund is intended to help develop an artificial intelligence approach to study the genetic factors related to Alzheimer’s disease. A team of researchers led by Zhongming Zhao, Ph.D., and Xiaoqian Jiang, Ph.D., both principal investigators and professors at McWilliams School of Biomedical Informatics in UTHealth Houston, are in the process of creating a deep-learning AI system. This system will link brain imaging with cell-specific genetic factors. To validate their AI models, the researchers will use neuroimaging and genetic data from Rush University Medical Center. They will also join the National Alzheimer’s Disease Sequencing Project AI/Machine Learning Consortium.
Zhao, who is also the director of the Center for Precision Health at McWilliams School of Biomedical Informatics, stated that this project will bridge the gap in Alzheimer's disease research between neuroimaging and genetic studies. Although there have been numerous computational analytical approaches published in each field, few can better address the link between neuroimaging and genetic data for a deeper understanding of the disease.
A significant amount of data related to molecular neuroimaging biomarkers and clinical information has already been generated in the context of Alzheimer's disease. However, researchers have not been able to connect many of the causal factors associated with the disease. To address this, scientists plan to use advanced machine-learning technology and an AI multimodality approach to group genetic and functional data. This will help to characterize the genetic risk of Alzheimer's disease. The researchers are calling this approach the "deep-learning brain" because it will focus on brain reading. The goal is to extend this model to the single-cell level, which will be called the "single-cell deep brain." This will allow for a more powerful way to dissect the genetic components of Alzheimer's disease.
To address the cognitive decline associated with Alzheimer's disease, researchers plan to integrate neuroimaging data into the deep-learning system. This will involve pairing distinct imaging features with genomic data to visualize their commonalities. Overall, the approach holds great promise for studying Alzheimer's disease and improving our understanding of this neurodegenerative disorder.
Researchers will use neuroimaging and genetic data from Rush University Medical Center, led by Christopher Gaiteri, PhD, assistant professor in the Department of Neurosciences, to validate their AI models. They will also collaborate with the national Alzheimer's Disease Sequencing Project AI/Machine Learning Consortium. The goal is to identify the link between genes and neuroimages to combine them into neuroimaging genetics, which can ultimately help explain the causes of cognitive decline in Alzheimer's disease. This understanding can help researchers and patients find better treatment options. The study's co-investigators are Paul Schulz, MD, a professor in the Department of Neurology at McGovern Medical School, and Kai Zhang, PhD, Yejin Kim, PhD, Yulin Dai, PhD, and Xiangning Chen, PhD, with McWilliams School of Biomedical Informatics. This research is funded by NIH grant U01AG079847.