AI hardware processing is going 3D, from square to cube, to boost processing power
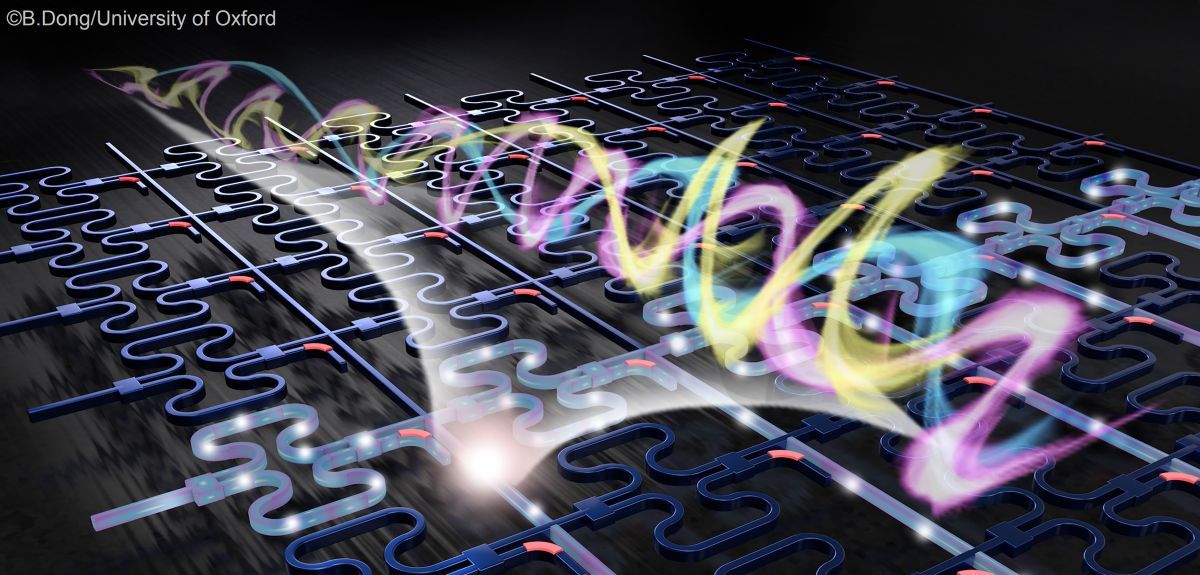
A team of researchers from the University of Oxford, in collaboration with other universities, has developed an innovative hardware system that combines photonic and electronic technologies to process 3D data. The system significantly enhances processing power for AI tasks. To test the hardware, the team analyzed 100 electrocardiogram signals simultaneously and achieved a 93.5% accuracy rate in identifying the risk of sudden death. The researchers believe that this approach could lead to a 100-fold increase in energy efficiency and compute density compared to current electronic processors if scaled up.
The efficiency of conventional computer chip processing doubles every 18 months. However, modern AI tasks require processing power that is currently doubling every 3.5 months. This means that new supercomputing paradigms are urgently needed to cope with this rising demand.
One possible solution is to use light instead of electronics to carry out multiple calculations in parallel using different wavelengths to represent different sets of data. In 2021, the same authors published groundbreaking work demonstrating a form of integrated photonic processing chip that could carry out matrix-vector multiplication at a much faster speed than the fastest electronic approaches. This breakthrough led to the creation of Salience Labs, a photonic AI company that emerged from the University of Oxford.
The team has now taken this concept further by adding an extra parallel dimension to the processing capability of their photonic matrix-vector multiplier chips. This higher-dimensional processing is made possible by using multiple different radio frequencies to encode the data, thereby achieving a level of parallelism that was previously impossible.
The team tested the hardware by applying it to the task of assessing the risk of sudden death from electrocardiograms of heart disease patients. They were able to successfully analyze 100 electrocardiogram signals simultaneously, accurately identifying the risk of sudden death with 93.5% accuracy.
The researchers estimated that even with a moderate scaling of 6 inputs x 6 outputs, this approach could outperform state-of-the-art electronic processors, potentially providing a 100-times enhancement in energy efficiency and compute density. The team anticipates further enhancement in supercomputing parallelism in the future by exploiting more degrees of freedom of light, such as polarization and mode multiplexing.
Dr. Bowei Dong, the first author of the publication, expressed his gratitude for the vibrant and collaborative platform provided by Oxford, which gave him the opportunity and courage to push the frontiers of advanced AI supercomputing hardware. Professor Harish Bhaskaran, the co-founder of Salience Labs and leader of this work, said that this is an exciting time to be doing research in AI hardware at the fundamental scale, and this work is one example of how what we assumed was a limit can be further surpassed.