LATEST
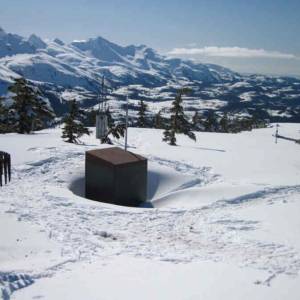
Enhancing water supply predictions through...
Wed, May 1st 2024Introduction: In a significant breakthrough, a...
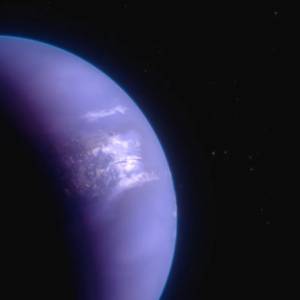
Unveiling the mysteries of distant worlds: NASA's...
Wed, May 1st 2024Introduction: In the vast expanse of the universe,...
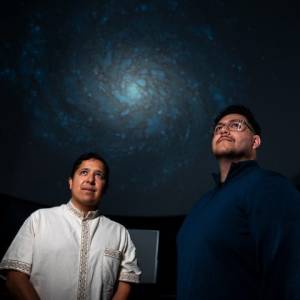
Shedding light on dark matter: Astronomers use...
Mon, Apr 29th 2024Introduction: The last century of scientific...
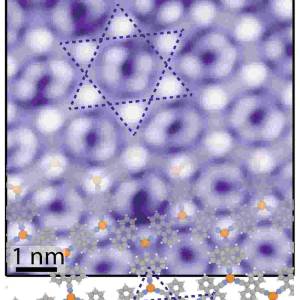
Switching a 2D metal-organic framework from...
Mon, Apr 29th 2024Introduction: In a remarkable achievement, an...
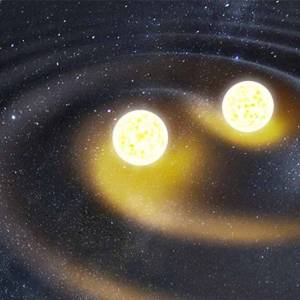
Researchers make progress in advancing...
Fri, Apr 26th 2024Researchers at the University of Minnesota Twin...