THE LATEST
Can machine learning make climate models relevant for local decision-makers?
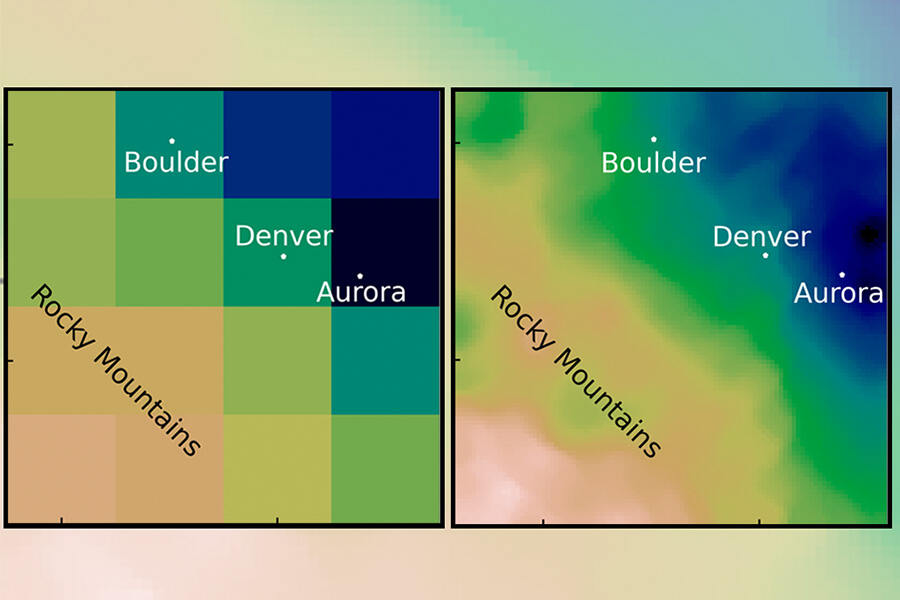
Researchers at MIT have proposed a method to make climate models more locally relevant using machine learning. They argue that this approach will reduce computational costs and speed up the simulations, making them applicable on smaller scales such as the size of a city. But one might wonder, can machine learning deliver on this audacious promise?
Climate models have long been a crucial tool in predicting the impacts of climate change. By simulating various aspects of the Earth's climate, scientists and policymakers can estimate factors like sea level rise, flooding, and temperature changes. However, these models have struggled to provide region-specific information quickly or affordably, limiting their usefulness on a local level.
The traditional approach to downscaling involves using global climate models with low resolutions and then generating finer details over specific regions. However, the lack of detail in the original low-resolution picture makes the downscaled version blurry and inadequate for practical use. Adding information through conventional downscaling methods, which combine physics-based models with statistical data, is not only time-consuming and computationally taxing but also expensive.
The MIT researchers claim to have found a solution in machine learning, specifically adversarial learning. Adversarial learning involves training two machines: one generates data, while the other judges the authenticity of the sample by comparing it to real data. The process aims to create super-resolution data, capturing finer details. The researchers simplified the physics going into the machine learning model and supplemented it with statistical data from historical observations.
While the concept of employing machine learning in climate modeling is not entirely new, the researchers admit that current approaches struggle to handle complex physics equations and conservation laws. By reducing the physics and enhancing the model with simplified physics and statistical data, the researchers assert that their approach produces similar results to traditional models at a fraction of the cost and time.
Another surprising finding was the minimal amount of training data required for the model. This efficiency in training allows for quicker results, with the potential to produce outputs within minutes, unlike other models that may take months to run.
The ability to run models quickly and frequently is vital for stakeholders such as insurance companies and local policymakers. The researchers argue that having access to timely information helps make decisions regarding crops, population migration, and other climate-related risks. However, the claim that this approach can quantify risk rapidly and accurately may still seem incredulous to many.
While the current model focuses on extreme precipitation, the researchers plan to expand its capabilities to include other critical events like tropical storms, high wind events, and temperature variations. They hope to apply the model to locations like Boston and Puerto Rico as part of their Climate Grand Challenges project.
Critics might question the extent to which machine learning can truly revolutionize climate modeling for local decision-making. Skepticism arises due to the complexities and uncertainties involved in climate science, as well as the potential limitations of simplifying physics models. The need for further research and verification of the model's performance before widespread adoption cannot be overlooked.
Although the MIT researchers express excitement about their methodology and the potential applications, it remains to be seen whether this machine learning approach can truly make climate models relevant for local decision-makers. Only time and rigorous scrutiny will determine the veracity of their claims and the full extent of the technique's capabilities.