THE LATEST
Chinese professor unveils an exciting vision for the advancement of intelligent supercomputing facilities
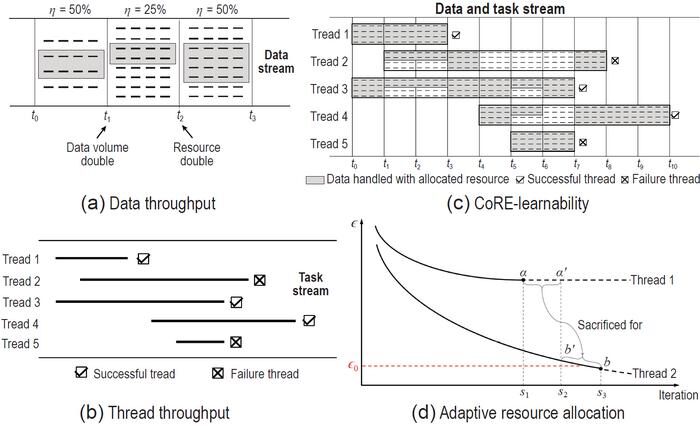
Enter the era of CoRE-learning
Have you ever thought about the untapped potential of computational resources in machine learning? Researchers at Nanjing University in China are exploring the impact of intelligent supercomputing facilities on machine learning performance. The groundbreaking research by Prof. Zhi-Hua Zhou introduces the concept of CoRE (COmputational Resource Efficient)-learning, which aims to utilize computational resources effectively.
Traditionally, the success of machine learning applications has been attributed to algorithms and data. However, Prof. Zhou emphasizes the often-overlooked influence of computational resources. In an attempt to bridge this gap, the CoRE-learning framework comes to light. This innovative approach introduces the concept of "machine learning throughput," allowing researchers to study the influence of computational resources on machine learning performance.
One key component of CoRE-learning is "time-sharing." This concept revolutionized computer systems, allowing them to serve multiple user tasks. Prof. Zhou observes that current intelligent supercomputing facilities tend to operate in an "elusive" style, where predetermined amounts of computational resources are allocated to specific tasks. This approach overlooks the possibility of wastage due to overallocation or the potential failure of a task due to underallocation.
CoRE-learning takes a step further by considering a task bundle—a collection of task threads during a specified period. Here, the importance of a "scheduling" mechanism becomes prominent, intelligently allocating resources to different task threads in real time. This sophisticated approach ensures not only hardware efficiency but also user efficiency, heralding a new era of intelligent supercomputing.
The CoRE-learning theoretical framework represents a breakthrough as it considers computational resource supply in machine learning performances within learning theory for the first time. By shedding light on power consumption reduction for training machine learning models, this research addresses a concerning issue for sustainable development. As the demand for machine learning grows, efficiency and resource optimization become increasingly crucial.
The findings, published in the National Science Review, pave the path for transforming the current "elusive" resource usage style in intelligent supercomputing facilities into a more efficient "time-sharing" style. This transition not only promises exciting advancements in machine learning but also holds the potential to reduce power consumption and contribute to sustainable development.
The groundbreaking research by Prof. Zhi-Hua Zhou and his team at Nanjing University promises a future where intelligent supercomputing facilities facilitate smarter resource allocation. As we embark on this journey, the fusion of CoRE-learning and cutting-edge technologies opens doors to unrivaled potential in the realm of computational resources.
Are we on the cusp of a new age of computing efficiency? Only time, and the curiosity of brilliant minds, will reveal the full extent of what lies ahead.