THE LATEST
University of Maryland makes dubious claims about its new AF2RAVE drug discovery method
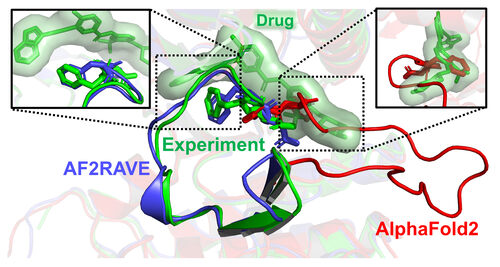
In a recent article published by the University of Maryland, scientists claimed that their newly developed AF2RAVE method is more effective than Google's AlphaFold 2 technology in the field of drug discovery. This groundbreaking method combines AlphaFold's AI prediction capabilities with physics-based simulations and aims to expedite the drug discovery process.
However, the claims made in the article raise skepticism and prompt a critical evaluation of the AF2RAVE approach. One key criticism is the lack of comprehensive data and independent validation of its performance. While the researchers emphasize a success rate of over 50% in matching drug candidates with existing drugs targeting specific protein shapes, the absence of detailed statistics and studies raises concerns about the credibility of their findings.
The skepticism surrounding the AF2RAVE method also stems from the challenges of predicting protein structures, especially non-native conformations. The complex nature of protein folding and the uncertainties in computational modeling raise questions about the feasibility of accurately predicting protein configurations solely based on AI and physics-based simulations.
Moreover, the competitive landscape of AI-driven drug discovery raises questions about the motivations behind such bold assertions and the potential impact on scientific integrity and public trust in cutting-edge research.
In conclusion, while the AF2RAVE method holds promise as a novel approach to drug discovery, rigorous validation, transparent reporting of results, and independent assessments are crucial to validating its efficacy and reliability. As the scientific community continues to explore the intersection of AI and physics in healthcare innovation, a critical and balanced evaluation of such claims is imperative to ensure meaningful progress in the field of drug discovery.
TRENDING
- Concerns raised over potential securities law violations by Supermicro
- Propelling the future of communications: Silicon chip innovation accelerates progress towards 6G networks
- NVIDIA's quarterly Data Center revenue hit a record $26.3 billion, marking a 16% increase from Q1 and a 154% increase from a year ago