THE LATEST
USC researchers develop an AI model that predicts the accuracy of protein-DNA binding
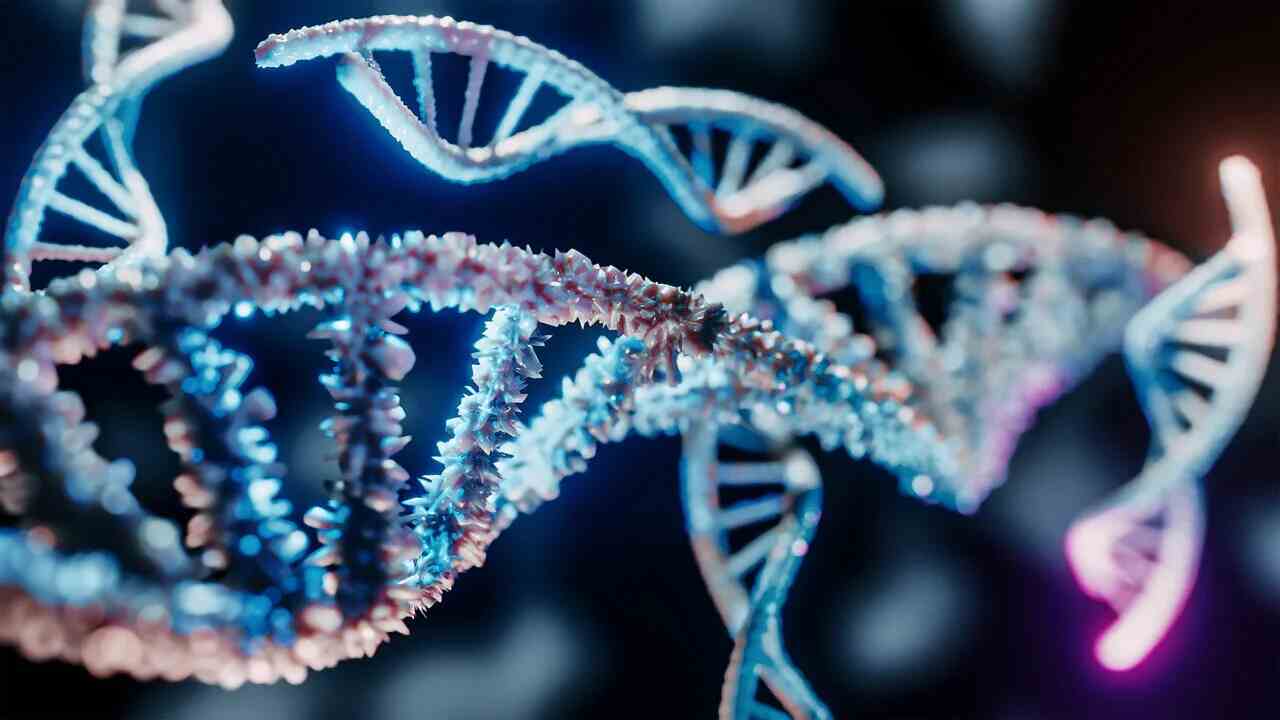
A groundbreaking development in the field of bioinformatics has emerged from the University of Southern California (USC). A team of researchers at USC has successfully developed an innovative artificial intelligence (AI) model that can predict the accuracy of protein-DNA binding with unprecedented precision. This achievement showcases the potential of AI to revolutionize the process of understanding protein-DNA interactions, offering promising prospects for discovering new drugs and medical treatments.
The newly engineered AI tool, Deep Predictor of Binding Specificity (DeepPBS), is a geometric deep learning model designed to forecast how various proteins might bind to DNA across different protein types. DeepPBS eliminates the need for time-consuming high-throughput sequencing or structural biology experiments by enabling scientists and researchers to input the data structure of a protein-DNA complex into an online computational tool.
Professor Remo Rohs, a pioneer in the field of Quantitative and Computational Biology at the USC Dornsife College of Letters, Arts and Sciences, emphasized the significance of DeepPBS in deciphering the intricacies of gene regulation. He pointed out that the tool's ability to predict protein-DNA binding specificity represents a fundamental shift, providing researchers with a versatile and efficient method that transcends the limitations of existing techniques.
This innovative AI model is built on geometric deep learning, a sophisticated machine-learning approach that leverages geometric structures to analyze data. By capturing the chemical properties and geometric contexts of protein-DNA interactions, DeepPBS generates spatial graphs that illustrate the complex relationship between proteins and DNA representations. Unlike conventional methods constrained to specific protein families, DeepPBS stands out by predicting binding specificity across diverse protein families, enabling researchers to explore novel avenues in protein design and manipulation.
The advent of DeepPBS marks a significant advancement in the realm of protein-structure prediction, complementing existing technologies like DeepMind’s AlphaFold that predict protein structures from sequences. USC's new AI tool serves as a complementary resource, especially beneficial for predicting binding specificity when experimental structures of proteins are unavailable. Its versatility extends the potential applications beyond drug development, encompassing advancements in cancer research, synthetic biology, and RNA studies.
The study, led by Professor Remo Rohs and a team of dedicated researchers from USC, alongside collaborators from other esteemed institutions, has been a pivotal step forward in the domain of bioinformatics. Supported primarily by the National Institutes of Health (NIH), this research has laid the groundwork for a transformative approach to predicting protein-DNA interactions, offering a glimpse into a future where AI accelerates scientific discoveries and medical breakthroughs.
In conclusion, USC's development of the DeepPBS AI model stands as a testament to the power of artificial intelligence in revolutionizing the field of bioinformatics. This achievement has the potential to reshape the landscape of protein-DNA binding specificity prediction, paving the way for innovative treatments, personalized therapies, and groundbreaking discoveries in the realms of medicine and biotechnology.