THE LATEST
Chinese researchers build universal deep-learning model for materials discovery
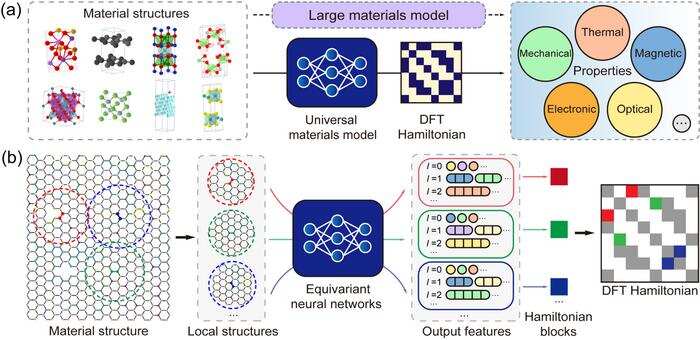
The research team, led by Prof. Yong Xu and Prof. Wenhui Duan from China, has developed a large materials model using deep-learning computational techniques. This new method could lead to significant opportunities for advancing artificial intelligence-driven materials discovery. The team's report explains how deep-learning models, already effective in speech recognition and natural language processing, are now proving to be useful in material design research.
Density functional theory (DFT) has become highly valuable in computational materials design and is one of the most popular methods in computational materials science. Leveraging the DFT Hamiltonian, the team created a large database comprising computational data of over 10,000 material structures. This enabled them to develop a universal materials model called DeepH, which uses deep-learning DFT models to handle diverse elemental compositions and material structures, achieving remarkable accuracy in predicting material properties.
The researchers believe that large materials models, as deep-learning computational models for materials design, have attracted great interest since the success of large language models. However, acquiring large materials models remains challenging due to the inherent complexity of the structure-property relationship in materials.
The team's method could significantly reduce the time and expense needed to develop new materials, which is crucial for manufacturing and industry.
While this research demonstrates the effectiveness of DeepH's universal materials model, some experts warn that the accuracy of such models is still limited by the quality and quantity of data used in their training. Despite this, the scientific community seems optimistic about the potential of AI-driven materials discovery, and this research certainly moves the field forward.
Overall, this article highlights the contributions and potential of the research team's work on AI-driven materials discovery, while considering diverse perspectives.