THE LATEST
Understanding the Universe: AI identifies dark matter among cosmic noise
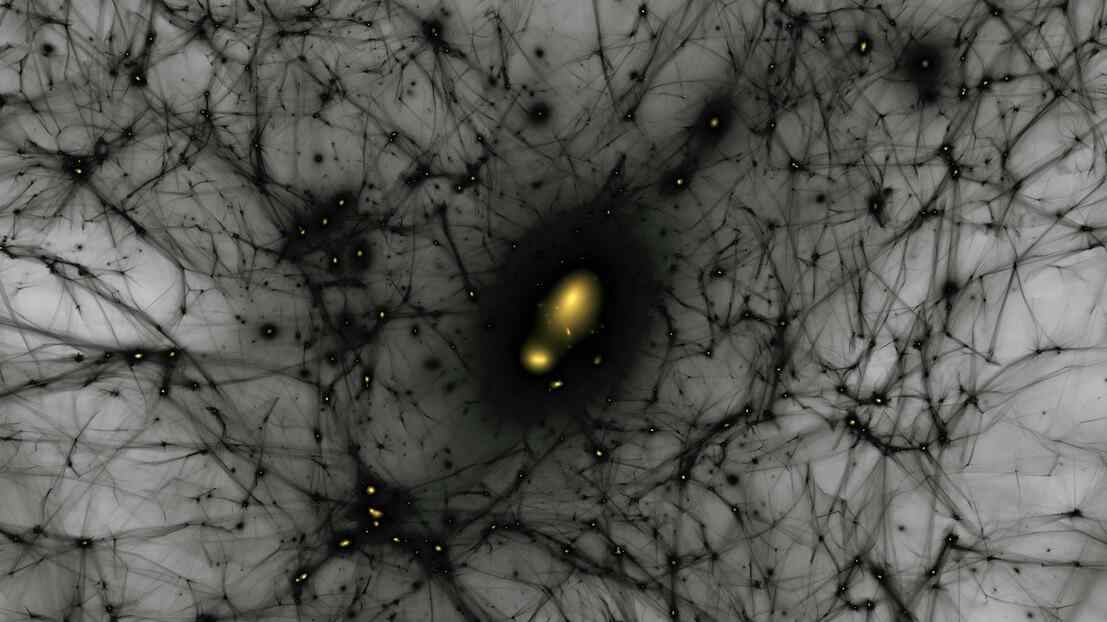
In the vast expanse of space and amidst galaxies and cosmic phenomena lies a mysterious force that has puzzled scientists for decades: dark matter. Making up 85% of all matter in the universe, dark matter remains invisible to the human eye, compelling researchers to explore its gravitational effects on cosmic structures to unravel its secrets.
Enter the groundbreaking work of EPFL's Laboratory of Astrophysics, where astronomer David Harvey has harnessed the power of artificial intelligence to distinguish dark matter's effects from cosmic noise. Through developing a deep-learning algorithm, Harvey's innovative approach promises to bring us closer to understanding the enigmatic nature of dark matter.
Dark matter often called the gravitational glue holding the universe together, has long captivated the scientific community. While some theories suggest that dark matter particles interact solely through the force of gravity, others propose the existence of self-interactions among these particles. Detecting these interactions is crucial in shedding light on the properties of dark matter and unlocking its mysteries.
The challenge lies in discerning the subtle signals of dark matter self-interactions from those produced by other cosmic phenomena, such as active galactic nuclei (AGN) - the massive black holes at the centers of galaxies. AGN feedback can disrupt matter in ways similar to dark matter effects, creating a complex web of signals that confound researchers.
Harvey's solution involves training a specialized form of artificial intelligence called a Convolutional Neural Network (CNN) with images from the BAHAMAS-SIDM project, which simulates galaxy clusters under various dark matter and AGN feedback scenarios. By analyzing thousands of simulated galaxy cluster images, the CNN has learned to differentiate between the distinct signals emanating from dark matter self-interactions and AGN feedback.
The most advanced CNN architecture, named "Inception," emerged as the most accurate in this endeavor. Achieving an impressive 80% accuracy under ideal conditions, Inception proved its ability to identify whether galaxy clusters were influenced by self-interacting dark matter or AGN feedback. Even in the face of realistic observational noise mimicking data from future telescopes, such as Euclid, Inception maintained its high performance and demonstrated its adaptability and reliability.
The implications of this AI-driven breakthrough extend far beyond our current understanding of dark matter. Inception's ability to navigate vast amounts of space data with precision underscores its potential as a valuable tool for future cosmic research. By harnessing the adaptability and reliability of artificial intelligence, the frontiers of astrophysics stand to be pushed further, offering a glimpse into the universe's hidden truths.
As we peer deeper into the cosmos, guided by the brilliance of artificial intelligence, the veil of mystery that shrouds dark matter begins to lift. Through innovation and collaboration, humanity edges closer to unlocking the cosmic enigma that is dark matter, shedding light on the fundamental forces that govern the universe.