THE LATEST
KAIST proposes innovative AI training method to revolutionize quantum mechanics calculations
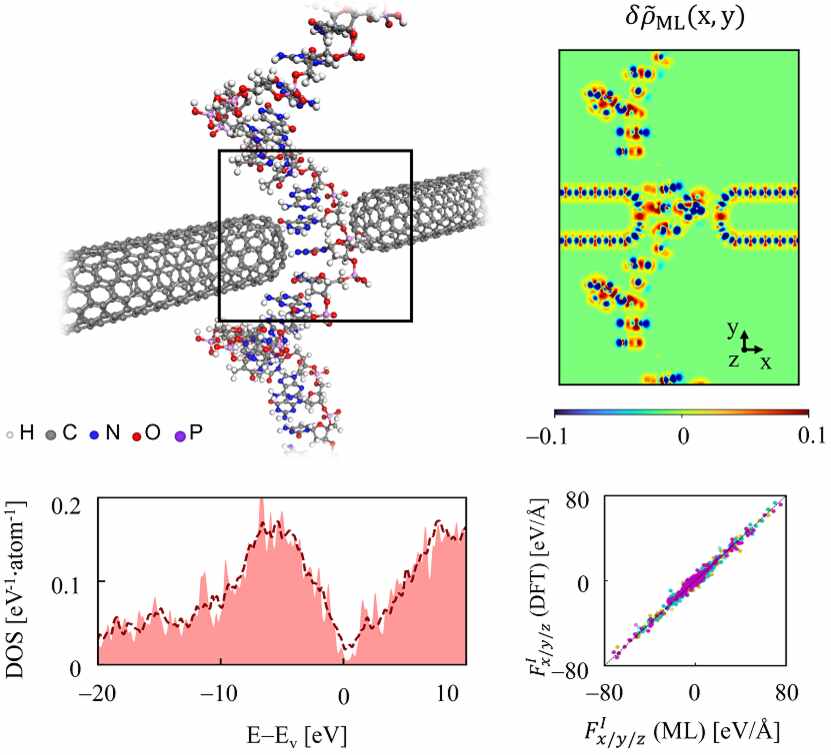
In a groundbreaking achievement, Professor Yong-Hoon Kim and his School of Electrical Engineering team at KAIST in South Korea have successfully accelerated calculations for electronic structure in quantum mechanics using a convolutional neural network (CNN) model. This pioneering approach not only represents a significant advancement but also has the potential to revolutionize the fields of next-generation materials and device design.
Integrating artificial intelligence (AI) with complex scientific computing has gained prominence, as evidenced by the recent Nobel Prizes in Physics and Chemistry awarded to scientists for their innovative use of AI in their respective fields. By significantly reducing computational time for intricate quantum mechanics simulations, the KAIST research team has set a new benchmark by predicting atomic-level chemical bonding information through a unique AI teaching method.
Traditionally, density functional theory (DFT) calculations in quantum mechanics, conducted using supercomputers, have been essential in various research and development domains, enabling fast and accurate predictions of quantum properties. However, the complexity of practical DFT calculations—requiring the generation of 3D electron density and the solution of quantum mechanical equations through a repetitive self-consistent field (SCF) process—has limited application to systems comprising a few hundred to a few thousand atoms.
Professor Kim's team challenged the conventional SCF process by developing the DeepSCF model. This model utilizes neural network algorithms to learn chemical bonding information in 3D space, significantly accelerating calculations. Their focus on electron density as a repository of quantum mechanical details, combined with a dataset of organic molecules, has validated and enhanced the efficiency of the DeepSCF methodology, even for large and complex systems.
This research breakthrough, led by Professor Yong-Hoon Kim and his team, signals a new era in material calculations utilizing artificial intelligence. The successful application of the DeepSCF methodology in predicting quantum mechanical and electronic structure properties marks a critical advancement that could reshape the landscape of computational materials science.
Ryong-Gyu Lee, a PhD candidate from the School of Electrical Engineering at KAwasle, is the first author of this research, which was recently published in Npj Computational Materials. The support of the KAIST Venture Research Program for Graduate and PhD Students and the National Research Foundation of Korea's Mid-Career Researcher Support Pro was instrumental in realizing this remarkable achievement.
With the convergence of AI and supercomputers heralding a new era in quantum mechanics calculations, KAIST's innovative approach underscores the institution's commitment to groundbreaking research that transcends boundaries and pushes the frontiers of scientific discovery. Only time will tell how this pioneering research will lead to transformative advancements in the fields of material science and computational engineering.