THE LATEST
AI model predicts multi-resistance in bacteria: Unveiling the power of big data
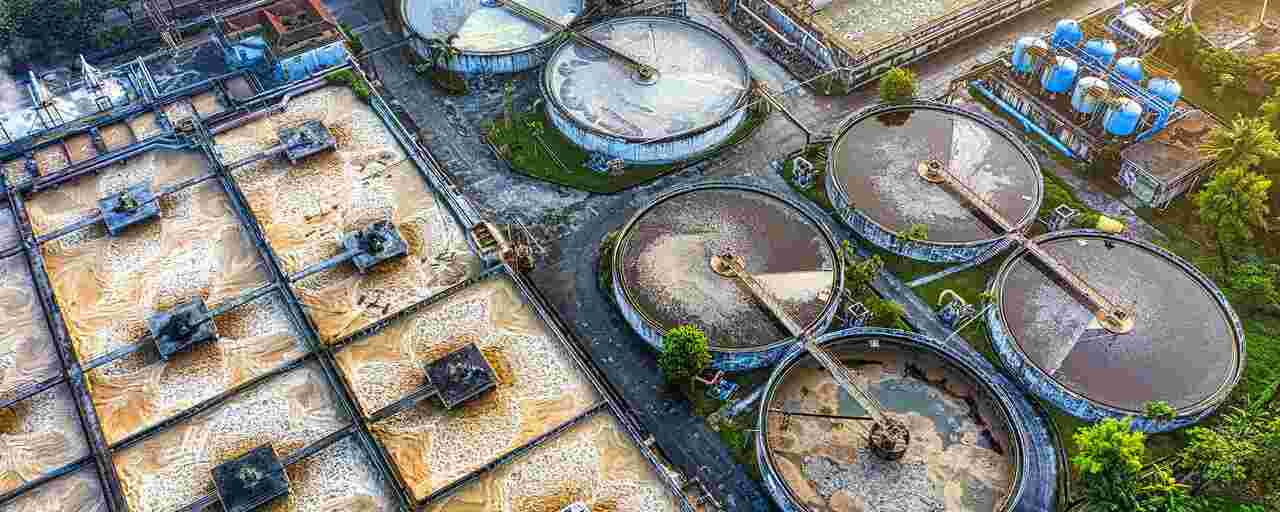
As antibiotic resistance poses a significant threat to global health, a groundbreaking study reveals a transformative approach to combatting this challenge. Researchers from Chalmers University of Technology, the University of Gothenburg, and the Fraunhofer-Chalmers Centre have utilized artificial intelligence (AI) to predict multi-resistance in bacteria, offering a promising pathway to fight against treatment-resistant bacterial infections.
The uniqueness of this study lies in its use of an extensive dataset that includes the genomes of nearly one million bacteria, a substantial compilation amassed by the global research community over many years. The research highlights the potential of AI to help us understand the complex biological processes that make bacterial infections challenging to treat.
Erik Kristiansson, a professor at the Department of Mathematical Sciences at Chalmers University of Technology and the University of Gothenburg, emphasizes the critical importance of deciphering the emergence of bacterial resistance to safeguard public health and enhance the healthcare system's ability to combat infections. The World Health Organization cites antibiotic resistance as one of the most significant threats to public health, complicating the management of diseases like pneumonia and sepsis.
Commenting on the research, David Lund, a doctoral student at Chalmers and the University of Gothenburg, highlights the extraordinary potential of AI and machine learning in addressing the complexities of bacterial infections. Lund states, "AI excels in navigating complex environments with vast datasets. Our study’s standout feature is the large volume of data used to train the model, showcasing AI’s ability to delineate the intricate biological mechanisms underlying bacterial resistance."
Through the AI model developed in this study, researchers can identify the environments where resistance genes are exchanged between bacteria, shedding light on the factors that increase the likelihood of gene swapping. Notably, bacteria found in humans and water treatment facilities are more likely to develop resistance through gene transfer, particularly when exposed to antibiotics.
The study highlights the role of genetic similarity among bacteria in facilitating gene transfer and lowering the metabolic cost associated with incorporating new genes. Kristiansson adds, "Our ongoing research aims to unravel the precise mechanisms governing this process, enhancing our understanding of how bacteria acquire resistance mutations."
The research team validated the model's predictive capabilities by accurately forecasting the transfer of resistance genes in four out of five instances where this occurred. This achievement underscores the potential of AI models to revolutionize molecular diagnostics, wastewater monitoring, and the identification of novel multi-resistant bacterial strains.
Looking ahead, the researchers hope to use AI models to swiftly detect the risks of resistance gene transfer to pathogenic bacteria and translate these insights into practical interventions. Kristiansson expresses optimism about future applications of AI and machine learning, envisioning a data-driven approach to unravel complex scientific questions and address emerging challenges in healthcare.
As we explore the realms of AI-driven insights and big data analytics, the possibilities for combating antibiotic resistance continue to expand. The intersection of cutting-edge technology and biological research invites us to explore new frontiers in disease understanding and treatment. How might the fusion of AI and extensive data repositories guide us toward a future where bacterial infections are no longer as daunting a challenge as they currently seem?