THE LATEST
Tulane researchers use AI to improve diagnosis of drug-resistant infections
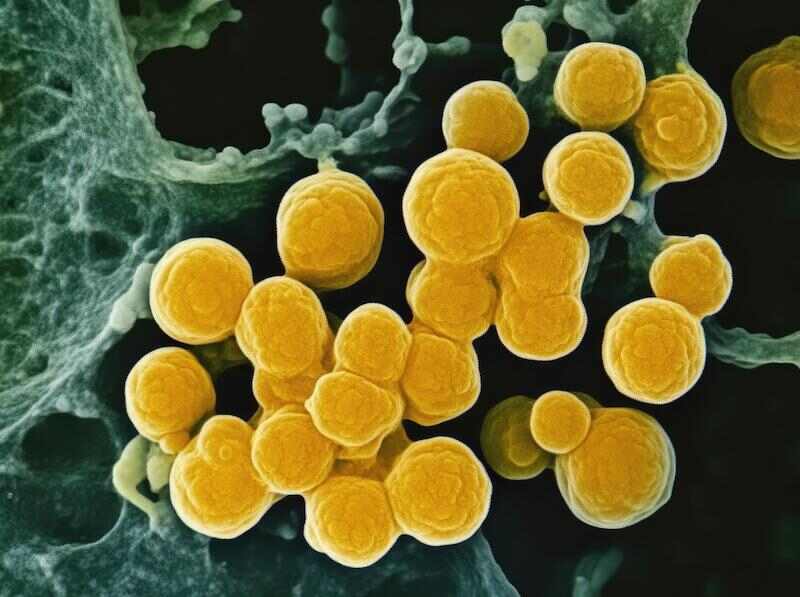
In a time when drug-resistant infections pose a significant threat to global health, the need for innovative solutions has never been more critical. Recent advancements in technology have provided a glimmer of hope, as researchers at Tulane University have introduced a groundbreaking artificial intelligence (AI)-based approach aimed at revolutionizing the diagnosis and treatment of these challenging infections.
The problem of drug-resistant infections, primarily driven by pathogens like tuberculosis and staphylococcus, continues to worsen, resulting in a serious healthcare crisis. The complexities involved—such as rising treatment costs and higher mortality rates—highlight the urgent need for advanced diagnostic tools. According to the World Health Organization, there were approximately 450,000 cases of multidrug-resistant tuberculosis in 2021, with success rates for treatment plummeting to just 57%.
To address this pressing issue, Tulane University scientists have developed an innovative AI-driven method designed to identify genetic markers of antibiotic resistance in notorious pathogens like Mycobacterium tuberculosis and Staphylococcus aureus. This cutting-edge approach introduces the Group Association Model (GAM)—a novel computational model enhanced by machine learning algorithms.
Unlike traditional diagnostic tools that often struggle to accurately determine resistance mechanisms, GAM represents a paradigm shift by analyzing the complete genetic profile of bacteria to identify the genetic mutations responsible for antibiotic resistance. Dr. Tony Hu, the Weatherhead Presidential Chair in Biotechnology Innovation and the director of the Tulane Center for Cellular & Molecular Diagnostics, describes this methodology as a way to uncover bacteria's resistance patterns without relying on preconceived notions.
The strength of GAM lies in its comprehensive analysis of whole genome sequences, allowing scientists to compare bacterial strains with varying resistance profiles. By identifying genetic changes that indicate resistance to specific drugs, this innovative model not only improves diagnostic accuracy but also reduces the occurrence of false positives, which can lead to inappropriate treatment decisions.
Additionally, GAM's integration with machine learning enhances its predictive capabilities, especially when dealing with limited or incomplete data. Validation studies conducted on clinical samples from China have shown that this advanced model significantly outperforms existing methods based on WHO guidelines in predicting resistance to critical front-line antibiotics.
Beyond its immediate applications in healthcare, the implications of this AI-powered diagnostic tool extend beyond medical laboratories. The potential to apply this methodology to other bacterial strains or even to agricultural contexts—where antibiotic resistance is increasingly problematic—underscores the versatility and impact of Tulane's pioneering research.
As lead author Julian Saliba aptly notes, combating drug-resistant infections requires a proactive approach. This innovative tool serves as a vital ally in this ongoing battle. By deepening our understanding of resistance mechanisms and facilitating early intervention, Tulane's novel AI-based method paves the way for personalized treatment regimens and heralds a new era in the fight against drug-resistant infections.