ACADEMIA
UMass Amherst computer scientist wins grant to better use large data sets generated by citizen science projects
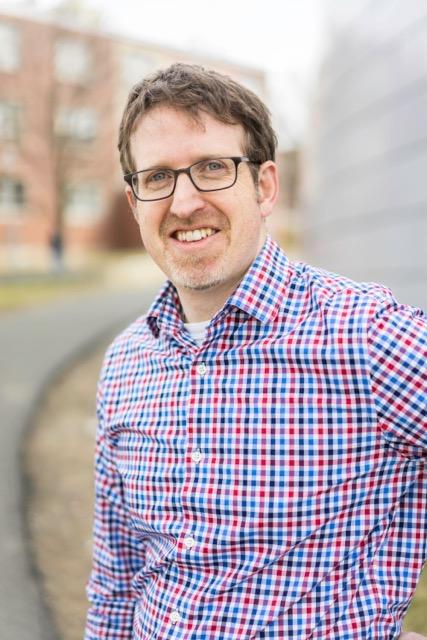
Computer science researcher Daniel Sheldon at the University of Massachusetts Amherst has been awarded a five-year, $550,000 faculty early CAREER grant from the NSF to design and test new algorithms and supercomputing approaches to help ecologists and other scientists better use large data sets generated by citizen science projects, animal tracking devices and earth observation instruments, among other goals.
As Sheldon explains, these new data sources hold "exceptional promise" for monitoring biodiversity, advancing scientific discovery and guiding decisions to conserve natural systems, but their full potential has not been realized in part because the information they provide is so diverse and varies over space and time, for example. "These qualities really challenge existing computational and statistical tools," he says.
One area he plans to address is related to what he calls "the explosion of data" coming from citizen science projects such as eBird. Sheldon has collaborated with eBird scientists, who collect observations from birdwatchers across the globe, since 2009. The researchers use big data methods to piece together observations to reveal complex patterns of bird occurrence and to guide international bird conservation efforts.
He says, "Traditionally, we only had data from surveys conducted once or twice a year, so we would never dream of modeling spring and fall migration. But now with huge amounts of evidence coming in every day of the year from people all over the continent, the data shows really clear evidence of migration patterns of different species across the continent."
"To really understand this behavior, we'd like to fit models," Sheldon adds. "With models we can make predictions and test hypotheses. For example, are migration routes changing? How does weather affect decisions about where and when to move? Where is mortality greatest for migratory birds? Answering these questions will improve both science and conservation."
"So, new data resources offer exciting possibilities to answer questions we could never ask before," he points out. But for each question there is a statistical model, and for each model there is a computational problem. As the number of variables in the model grows and the relationships between them get more complex, the computation can become very, very challenging, he adds.
"Yet we want to solve these problems because the payoff is really interesting," says the algorithm expert. "We'll be working on this grant to develop efficient algorithms for complex models. At the highest level, I hope we'll lay a really broad computational foundation for a few key problems in handling the new abundance of data. I want to help convert it into scientific knowledge and actionable decisions for conservation."
His CAREER grant, NSF's highest award to junior faculty, will also support interdisciplinary collaborations and development of a new class of mathematical models called "probability generating function networks" with efficient algorithms for reasoning about populations.
He explains, "A basic problem in ecology is modeling animal populations from survey data where not every individual is observed. This means reasoning about a hidden quantity, the true population size. But we don't know ahead of time how big the population could be, so there are an infinite number of possible values. This turns out to be a barrier to basic probabilistic inference algorithms, which enumerate every possible value. We are developing methods to fix this by being more clever about how you structure the computation."
Another thread of investigation will focus on causal reasoning about citizen scientist data, which encodes information about animal populations but may also have systematic bias. For example, observers have different skill levels, and make their own decisions about when and where to observe animals. The causal reasoning will learn to recognize and correct systematic errors that come from the observation process, and will improve overall data quality.
Another aspect of his study will be to develop algorithms to optimize decision-making in the presence of multiple objectives. These will help balance many different interests, or "make the best possible tradeoffs," Sheldon notes.
For example, such algorithms can be used to maximize the amount of power obtained from building new dams in a river basin while minimizing barriers to fish and other organisms. "We can develop algorithms that will help you achieve optimal tradeoffs in making such decisions," he says.