ACADEMIA
Artificial intelligence is paving the way for precision medicine
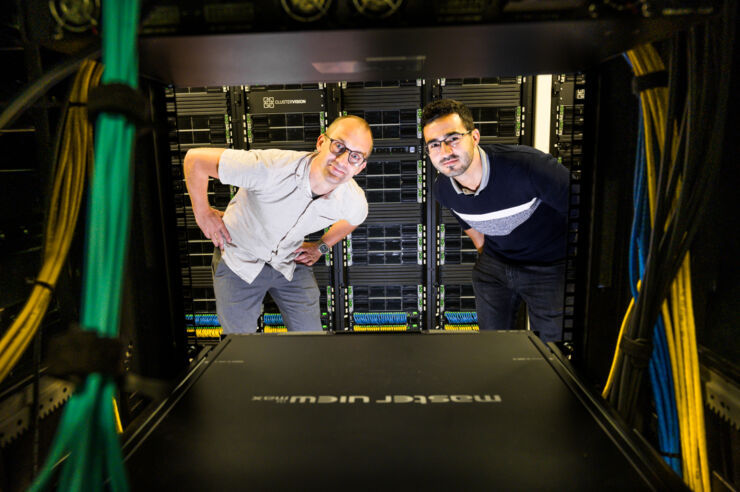
Artificial Intelligence (AI) models can accurately estimate a person's age and determine whether they have a smoking history or not. Researchers at Linköping University have developed an AI-based method that can be used to address various medical and biological issues. This method can identify epigenetic markers that were previously known and those that are new and associated with conditions. The goal of AI models is to simplify complex biological data and extract the most relevant characteristics and patterns. The ultimate objective is to create an interpretable AI model that can help understand why someone is ill or not.
Epigenetics refers to the regulation of gene activity, which can be compared to a power switch that turns genes on or off without altering them. This process can be influenced by several factors, such as smoking, dietary habits, and environmental pollution. To develop personalized treatments and preventive strategies, researchers at Linköping University (LiU) have trained numerous AI neural network models using epigenetic data from over 75,000 human samples. These models are of the autoencoder type, which helps to self-organize the data and identify interrelation patterns in the vast amount of information.
Research conducted by LiU scientists has revealed that smoking leaves permanent traces on the DNA even after a person quits smoking. The researchers developed a model that compared the effects of smoking on the body with existing models. These models are based on specific epigenetic changes that occur in the lungs as a result of smoking. The new model can detect if someone is a current, former, or non-smoker. Additionally, other models that utilize epigenetic markers can estimate an individual's chronological age or group of individuals based on their health status.
The researchers at Linköping University trained an autoencoder and used its results to classify individuals on age, and smoker status and diagnose the disease systemic lupus erythematosus, SLE. While existing models depend on selected epigenetic markers, the autoencoders developed by the researchers performed equally well or better. The researchers discovered that their models could identify new markers associated with the condition they were examining, such as markers for respiratory diseases and DNA damage. The autoencoder models were designed to compress complex biological data into a representation of the most relevant characteristics and patterns in the data. The researchers allowed the data to speak for itself, and the autoencoder self-organized the data in a way similar to how it works in the body. Using the most important characteristics found by the autoencoder, the researchers can create models to classify a large amount of environment-related, individual-specific factors where there is not enough training data for complex AI models.
It is sometimes difficult to understand how certain types of AI work. They are like black boxes that provide answers but it is unclear how they arrived at those answers. However, Mika Gustafsson and his team are working on creating interpretable AI models. These models let researchers look inside the black box and better understand how the AI works. This is important because it helps us understand why certain conditions and diseases occur, not just whether someone is affected or not. The research was funded by several organizations including the Swedish Research Council, the Wallenberg AI, Autonomous Systems and Software Program (WASP), and the SciLifeLab & Wallenberg National Program for Data-Driven Life Science (DDLS).