ACADEMIA
New research conducted by Concordia University suggests that better wind speed predictions could be beneficial for urban power generation
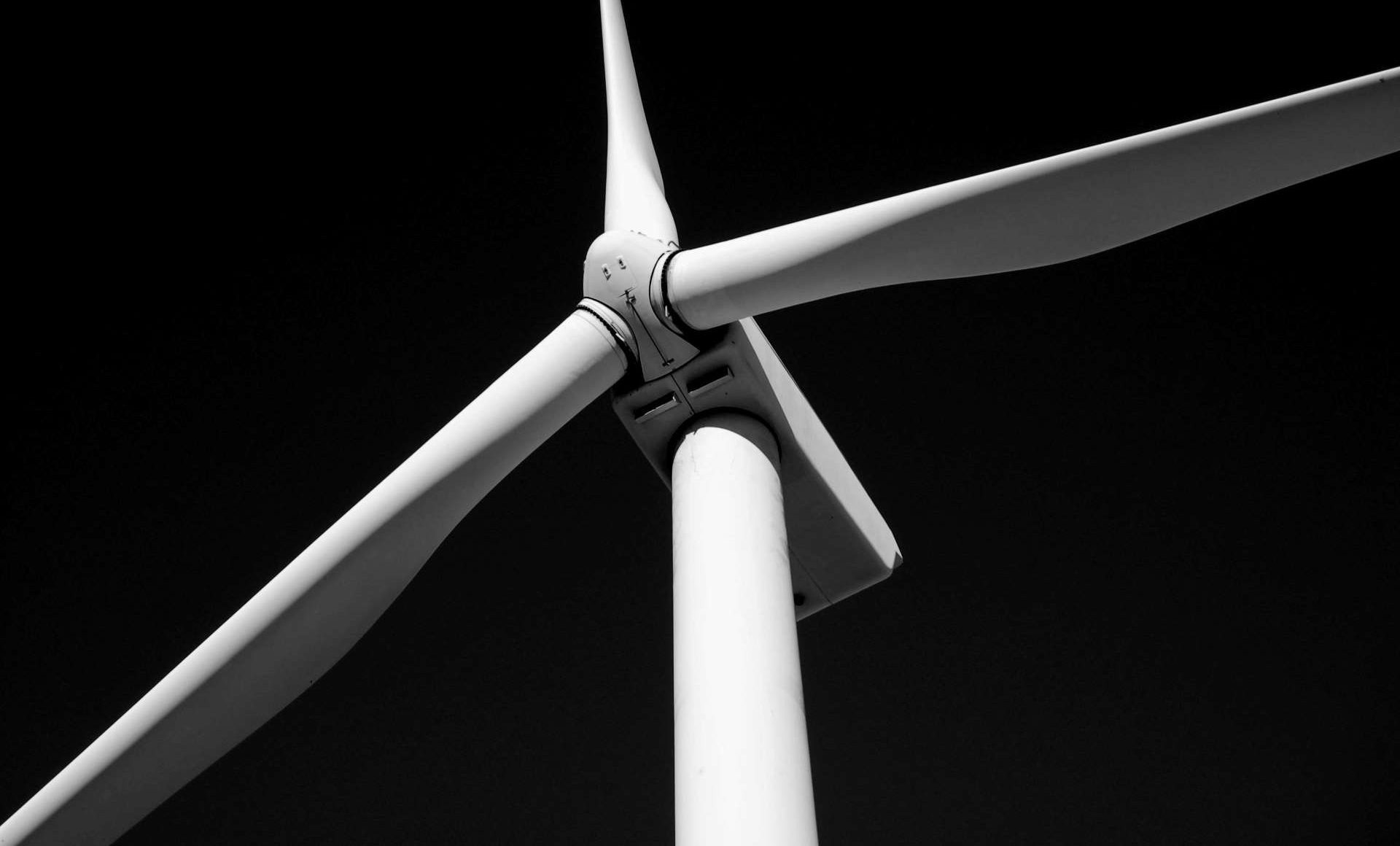
In today's world, where renewable energy is gaining importance, wind-generated electricity is expected to play a vital role in powering our cities. However, accurately predicting wind speed has always been a challenge. Researchers at Concordia University have developed a hybrid method that integrates multiple models, which has improved the accuracy of wind speed forecasts. This groundbreaking research has wide-ranging implications for urban power generation and the transition towards sustainable energy sources.
Challenges in Predicting Wind Speed:
Wind speed is a critical parameter in estimating wind energy potentials in a given location. Reliable wind speed forecasts are essential for utilities to effectively harness wind power and balance the grid. Although several models exist to predict wind speed, they vary in accuracy and reliability. These models often struggle to capture the stochastic behavior and fluctuations of renewables, making it challenging for utilities to design and operate microgrids efficiently.
The Concordia Hybrid Method:
The Department of Building, Civil, and Environmental Engineering at the Gina Cody School of Engineering and Computer Science led the Concordia study, which proposes a hybrid method that combines the strengths of different models. The researchers integrate data analysis and outputs from a Weibull probability distribution and a numerical weather prediction (NWP) model.
The Weibull distribution predicts wind speed probabilities based on historical data and other variables, while the NWP uses physical principles and a complex algorithm to simulate future behavior. By combining these models, the researchers were able to significantly improve the accuracy of wind speed forecasts, reducing forecasting errors by up to 30%.
The Impact and Findings:
Initially, the study fused Weibull probabilities into a Long Short-Term Memory (LTSM) model, a powerful recurrent neural network suitable for time-series analysis. The results were already promising, but the addition of data from the NWP further enhanced the predictive capabilities of the model. Compared to non-hybridized LTSM predictions, errors in wind speed forecasting over a 48-hour horizon were reduced by 32%.
Looking Ahead:
As wind power continues to grow globally, accurate wind speed prediction is crucial for achieving sustainable energy goals. According to the International Energy Agency, generating 7,400 TWh from wind alone by the end of this decade is necessary to reach the net-zero emissions target by 2050. Therefore, investing in advancements like the Concordia hybrid method is vital to meet these targets and ensure a smooth transition to renewable energy sources.
Concordia's Commitment to Decarbonization:
The research carried out by the Concordia team aligns with the university's commitment to decarbonization and its goal of achieving Net Zero Emissions by 2050. By diversifying energy sources and establishing local capacities, Concordia aims to reduce reliance on the vulnerable existing grid and enhance operational efficiency during power outages.
Conclusion:
Wind speed prediction is a crucial aspect of harnessing wind energy for urban power generation. The innovative hybrid method developed by Concordia researchers offers a significant advancement in accurately forecasting wind speed. By integrating the strengths of different models, the Concordia team was able to improve forecasting accuracy by up to 30%. As renewable energy becomes increasingly important in addressing climate change, research like this plays a vital role in making our energy systems more sustainable and efficient. Concordia's commitment to decarbonization and the development of innovative solutions positions the university as a Canadian leader in the transition toward a cleaner and greener future.