GOVERNMENT
Patkar’s new supercomputational framework shows shifting protein networks in breast cancer may alter gene function
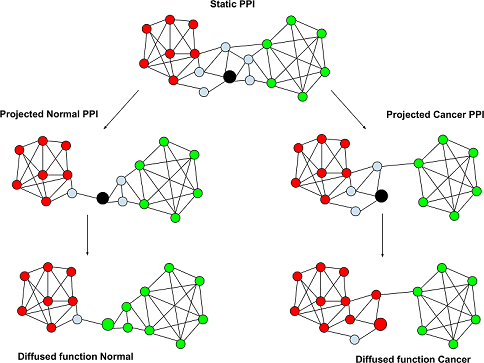
Changes in gene function in tumor samples correlate with patient survival
A given gene may perform a different function in breast cancer cells than in healthy cells due to changes in networks of interacting proteins, according to a new study published in PLOS Computational Biology.
Previous research has shown that a protein produced by a single gene can potentially have different functions in a cell depending on the proteins with which it interacts. Protein interactions can differ depending on context, such as in different tissues or developmental stages. For instance, one protein produced by a key fruit fly gene serves two separate functions over the course of fly development.
Building on this concept, Sushant Patkar of the University of Maryland and colleagues hypothesized that alterations in protein interaction networks in breast cancer cells may change the function of individual genes. To test this idea, they analyzed protein expression in 1,047 breast cancer tumors and 110 healthy breast tissue samples, using data from The Cancer Genome Atlas project.
The researchers developed a supercomputational framework to determine the structure of protein interaction networks in each sample and infer which genes performed different cellular functions within these networks. Then, they compared the number of genes inferred to perform each function in cancer cells relative to healthy cells.
The analysis revealed that several functions were associated with more or fewer genes in cancer cells than in healthy cells, but not because of changes in the expression of these genes. Instead, their function changed due to changes in their protein interaction networks. The researchers also showed that profiling a patient's tumor tissue according to these functional shifts served as an effective method to predict their cancer subtype and their survival.
"While it is completely plausible for a gene to lose or acquire novel biological functions, examples of such changes have predominantly been observed in the context of evolution," Patkar says. "We have developed a bioinformatics approach that suggests that such changes might alternatively occur through changes in the interactions of proteins encoded by the gene."
Next, the team plans to validate their supercomputational framework as a tool to assess changes in gene function in other biological contexts, such as in other diseases and tissues.
Access to the freely available article in PLOS Computational Biology: http://journals.plos.org/ploscompbiol/article?id=10.1371/journal.pcbi.1005793