INDUSTRY
Fremling's SNIascore identifies 1000 supernovae
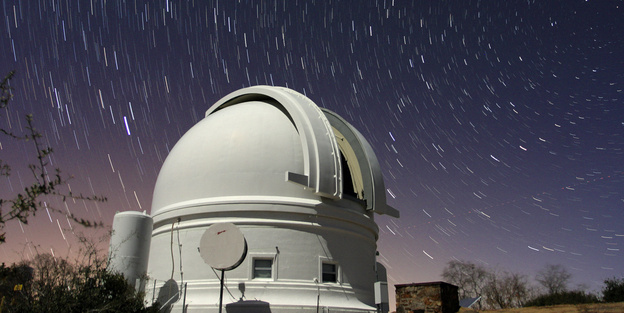
Today’s astronomical facilities scan the night sky deeper and faster than ever before. Identifying and classifying known and potentially interesting cosmic events is becoming impossible for one or a group of astronomers. Therefore, increasingly they train supercomputers to do the work for them. Astronomers from the Zwicky Transient Facility collaboration at Caltech have announced that their machine-learning algorithm has now classified and reported 1000 supernovae completely autonomously.
“We needed a helping hand and we knew that once we train our computers to do the job, they would take a big load off our backs”, says Christoffer Fremling, a staff astronomer at Caltech and the mastermind behind the new algorithm, dubbed SNIascore. “SNIascore classified its first supernova in April 2021 and a year and a half later we are hitting a nice milestone of 1000 supernovae without any human involvement.”
Many of the current and most exciting scientific questions that astronomers are trying to answer require them to collect large samples of different cosmic events. As a result, modern astronomical observatories have become relentless data-generating machines that throw tens of thousands of alerts and images at astronomers every night. This is particularly true in the field of time-domain astronomy, in which researchers look for fast-changing objects, or transients, such as exploding and dying stars known as supernovae, black holes eating orbiting stars, asteroids, and more.
“The traditional notion of an astronomer sitting at the observatory and sieving through telescope images carries a lot of romanticism but is drifting away from reality,” says Matthew Graham, the ZTF project scientist at Caltech.
Apart from freeing time for astronomers to pursue other science questions, the machine learning algorithm is much faster at classifying potential supernova candidates and sharing the results with the astronomical community. With SNIascore the process is shortened from 2-3 days to about 10 min, or near real-time. Such early identification of cosmic explosions is often critical to better study their physics.
“SNIascore sits on top of other underlying machine learning algorithms and layers that we have developed for ZTF, and it demonstrates well how machine learning applications are coming of age in near real-time astronomy,” says Ashish Mahabal, a computational scientist at Caltech’s Center for Data-Driven Discovery who leads machine learning activities for ZTF.
For now, SNIascore can only classify what’s known as Type Ia supernovae, or the “standard candles” in the sky used by astronomers to measure the universe's expansion rate. These are dying stars that go bang with a thermonuclear explosion of consistent strength. However, Christoffer and colleagues are working hard on extending the capabilities of the algorithm to classify other types of supernovae in the near future.
SNIascore is currently adapted to work with the SEDM spectrograph (Spectral Energy Distribution Machine) housed in a dome just a few hundred feet away from the ZTF camera at the Palomar Observatory. ZTF scans the sky continuously and sends hundreds of thousands of alerts every night for potential cosmic transients to astronomers around the world. The SEDM spectrograph is triggered to follow up and observe the most promising ones. It produces a spectrum of the cosmic event which carries information about the intensity of various frequencies of the light caught by the telescope camera. This spectrum is what can tell astronomers definitively what kind of event is being observed. Using clever machine learning techniques, Christoffer’s team has trained SNIascore to read the SEDM spectra remarkably well.
“SNIascore is incredibly accurate. After 1000 supernovae we have seen how the algorithm performs in the “real world” and we have had no clear misclassification since launching back in April 2021. This gives us the confidence to go ahead and implement the same algorithm with other observing facilities,” added Fremling.
He and colleagues are currently adapting SNIascore to work with the upcoming SEDMv2 spectrograph mounted on the 2.1m telescope at the Kitt Peak Observatory in Arizona. SEDMv2 will be the advanced version of SEDM and will allow for fainter supernovae to be detected and classified. Currently, SNIascore classifies on average two supernovae every night. With SEDMv2 this number can potentially double.
The advantages of SNIascore go beyond quickly and reliably building large datasets of supernovae. Astronomers looking for other transient events can now quickly rule out candidates classified by the SNIascore as supernovae such that no telescope time is wasted on following them when the search is for other types of cosmic explosions.
Other efforts for classifications of transient events also use machine learning but they rely only on the so-called “light curve” of the event or the amount of light seen by the telescope as an evolution of time. SNIascore has the advantage of being trained on and using spectroscopic information, the only robust way to confirm the nature of most transients. The algorithm is open source and other groups can adapt it to their own telescope facilities.
“The most challenging part in implementing SNIascore was to train the algorithm. It required that humans carefully check images and build an impeccable training dataset. After 1000 automatically classified supernovae, looking back I think it was entirely worth the effort,” says Fremling.
The SNIascore was developed as part of ZTF’s Bright Transient Survey - currently, the largest supernovae survey available to the astronomical community. The entire BTS dataset has close to 7000 supernovae 90% of which were discovered and classified by ZTF (10% were contributions from other groups and facilities).
“Our ambition is to continue to grow the BTS dataset with the help of SNIascore in the future to build the most comprehensive sample of supernovae which astronomers can use to answer fundamental questions in cosmology such as how fast the universe is expanding and to potentially map the dark matter distribution and the large scale structure of the universe,” added Fremling.