PHYSICS
China uses ML method for reconstructing high-resolution ocean subsurface salinity dataset
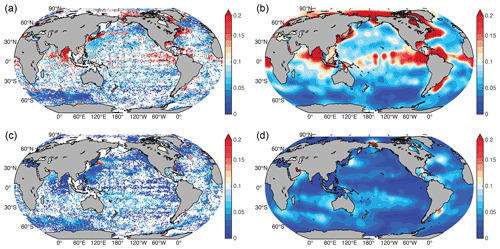
As a key parameter of seawater, salinity plays a vital role in regulating ocean density, stratification, and circulation. It also indicates the coupling between the ocean, atmosphere, and land through the water cycle. Gridded ocean datasets with complete global ocean coverage are significant to marine and climate research.
Currently, owing to the sparsity of in situ observations, most ocean salinity gridded products are with 1° × 1° horizontal resolution, which is difficult to meet the requirements of small-scale marine information research.
Recently, researchers from the Institute of Atmospheric Physics (IAP) of the Chinese Academy of Sciences have reported a high-resolution (0.25° × 0.25°) ocean subsurface (1–2000 m) salinity dataset for the period 1993–2018 using a machine-learning method called feed-forward neural network.
The study was published in Earth System Science Data on Nov. 18.
The study merges in-situ salinity profile observations with high-resolution (0.25° × 0.25°) satellite remote sensing altimetry absolute dynamic topography, sea surface temperature, sea surface wind field data, and a coarse resolution (1° × 1°) gridded salinity product.
"IAP1° gridded salinity dataset was formally released in 2020. Two years later, we developed the new 0.25° × 0.25° reconstruction dataset, or what we call IAP0.25°," said Prof. CHENG Lijing, corresponding author of the study.
Compared with the available IAP 1° × 1° resolution product, the new dataset shows more realistic spatial signals in regions with strong mesoscale variations, e.g., the Gulf Stream, Kuroshio, and Antarctic Circumpolar Current regions. "This indicates the efficiency of the machine learning approach in bringing satellite observations together with in-situ observations," said Prof. CHENG.
According to the study, the large-scale salinity patterns from IAP0.25° are consistent with the IAP1° gridded salinity field, suggesting the persistence of the large-scale signals in the high-resolution reconstruction. The SHAP method is also used to evaluate the effects of different inputs on the reconstruction of IAP0.25°.
The new IAP0.25° dataset is available at http://doi.org/10.57760/sciencedb.o00122.00001, http://www.ocean.iap.ac.cn/ftp/cheng/IAP_v0_Ocean_Salinity_0p25_FFNN_0_2000m/, and http://www.ocean.iap.ac.cn/.